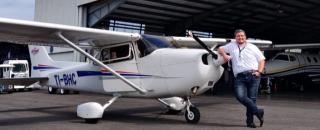
Understanding Fine-Tuning
Fine-tuning is the process of further training an existing language model on your specific data. Think of it like customizing a highly skilled professional for your specific industry - possible, but requiring significant expertise, resources, and data to do well.
When to Consider Fine-Tuning:
Fine-tuning makes most sense when:
- You have a large, high-quality dataset (typically thousands of examples).
- Your use case requires deep domain expertise.
- You tried simpler solutions like prompt engineering or RAG haven't provided adequate results.
- You have the technical expertise and resources for ongoing model maintenance.
When to Consider Alternatives:
Often, simpler approaches can achieve your goals:
- RAG for incorporating current or company-specific information
- Prompt engineering for adapting model behavior
- Zero-shot learning for handling new tasks without training
- Few-shot learning for quick adaptation with minimal examples
The Future of Model Customization
As language models evolve, the need for fine-tuning may decrease for many use cases. New techniques like parameter-efficient fine-tuning (PEFT) and instruction tuning are making models more adaptable with less overhead.
Remember: The goal isn't to have a fine-tuned model - it's to solve your business problems effectively. Choose the simplest approach that meets your needs, let's review the following example on building your own self-hosted ChatGPT Clone:
Ready to explore how fine-tuned AI can transform your enterprise applications? Experience Appsmith's AI platform and start building customized AI solutions that speak your business language.